Challenges and Opportunities for AI Data Center and Cooling Solution
The threat of artificial intelligence (AI) has been a constant theme in science fiction for decades. On-screen villains, such as the robots in The Matrix, have stood against humanity, forcing it to overcome the threats posed by these technologies.
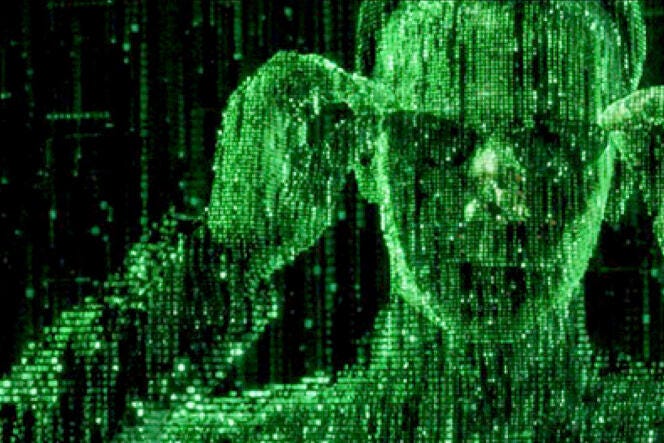
Interest in the potential of Artificial Intelligence (AI) and Machine Learning (ML) in real-world applications continues, and new algorithms, applications and software keeps popping up. Recently, ChatGPT evoked great deal of interest of the public in what AI can do and has sparked discussions about how AI will affect the nature of work and learning. Millions of users around the world are already using ChatGPT, Bard and other AI interfaces, but what most users don’t realize is that the communications with AI assistants don’t exist without the support of large data centers around the world.
Efficient Training
Enterprises are investing in AI clusters within their data centers to begin building, training, and refining AI models to fit their business strategies. These AI cores are in consist of GPUs (Graphics Processing Units) where they provide the powerful parallel processing power that AI models need to train algorithms. GPUs are best at parallel processing and are well suited for AI. This diagram below illustrates the role of the CPU and the GPUs in the Smart Computing Center.
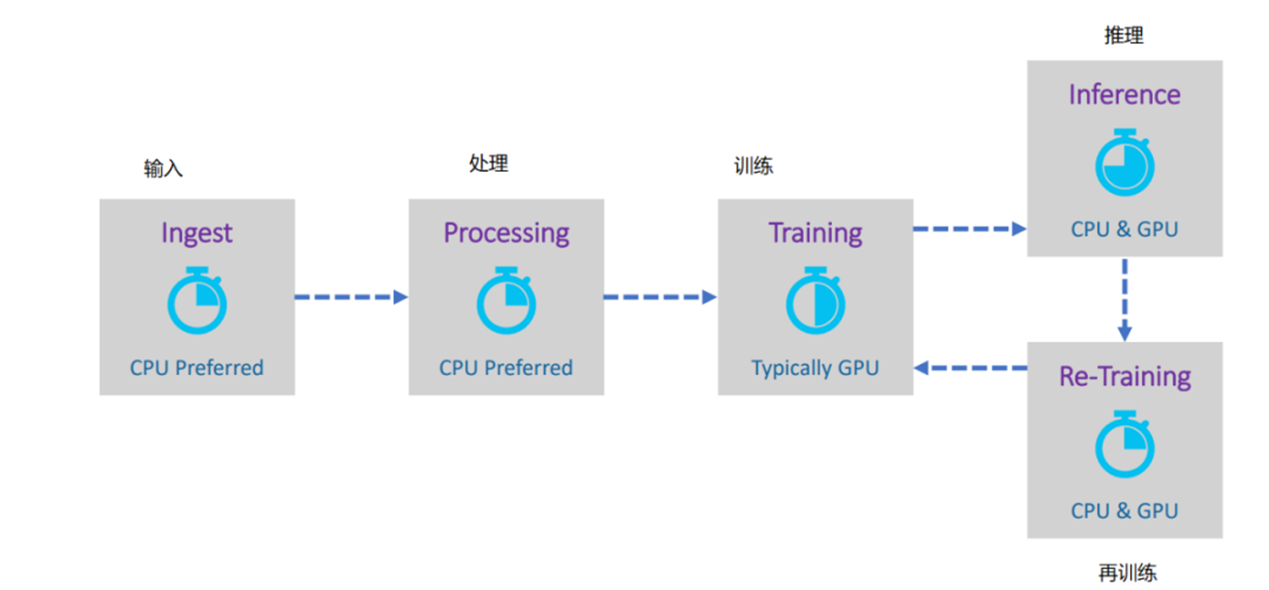
Through the process of importing datasets, training, and learning, AI analyzes the data and learn to understand and process information. For example, training based on the common features that distinguish cats from dogs determines whether the figure is a cat or a dog. The generative AI then processes the data to create an entirely new image or text.
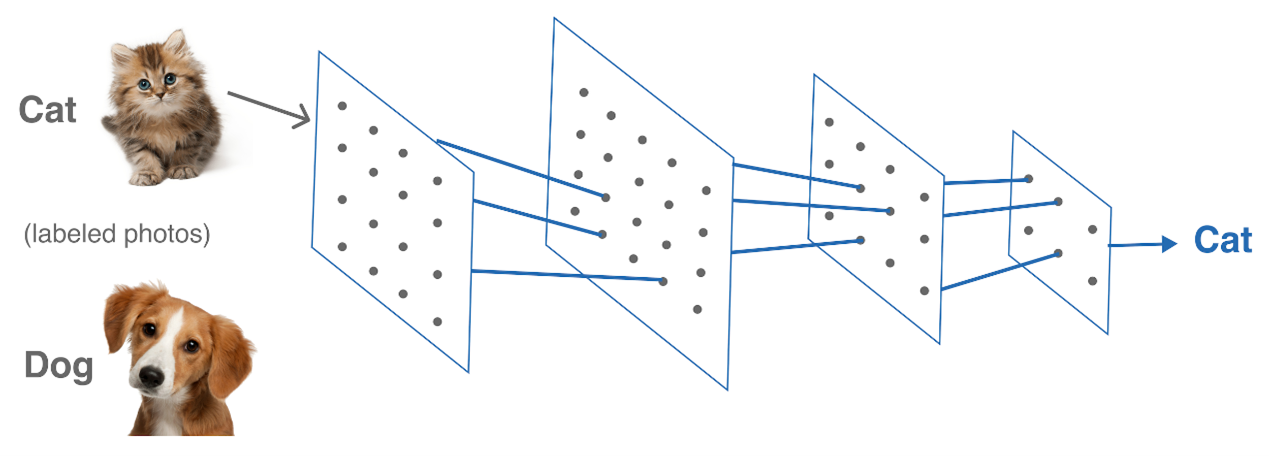
While this kind of “smart” processing has attracted a great deal of interests around the world, developing AI system is both costly and energy-intensive, as it requires large amounts of data for training. These models for training and running AI are more than a single machine can handle, and AI models grow in Peta FLOPS (floating point operations per second). Multiple GPUs on many servers and racks work together to be able to handle these large models, and these compute clusters are maintained in data center to work collaboratively to process the data. These GPUs must be connected via a high-speed connection to do the work of AI. The quantity of GPU equipped in each rack space is limited by energy consumption and cooling capacity of the GPUs, so the physical layout must be optimized, and link latency minimized.
This is where immersion cooling comes into play. According to a recent study, on average, servers and cooling systems account for the most significant shares of direct electricity use in data centers, followed by storage drives and network devices.
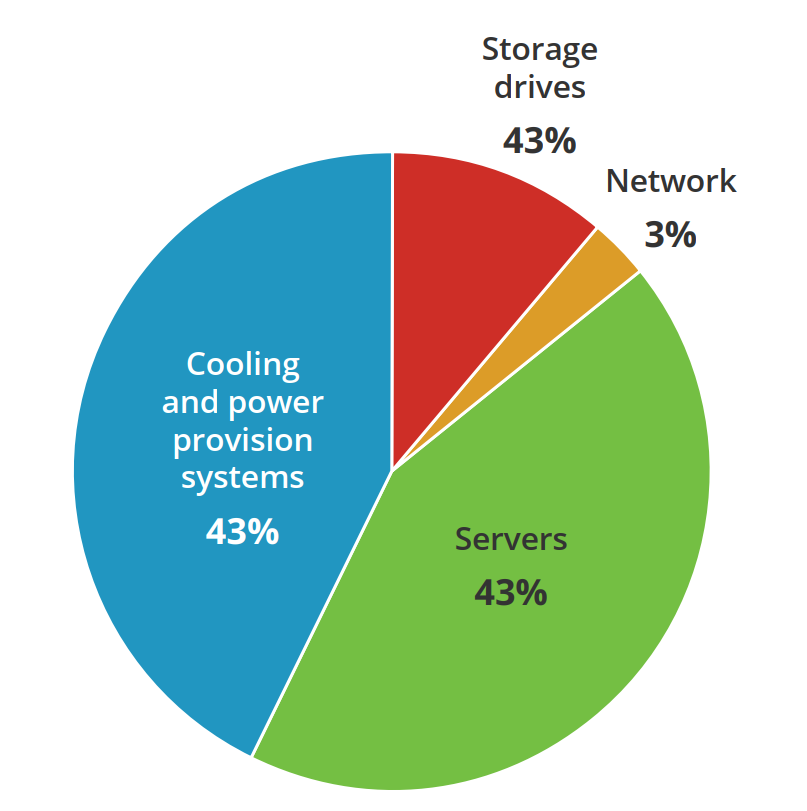
Some of the world’s largest data centers can contain tens of thousands of IT devices and require over 100 megawatts (MW) of power capacity to power around 80,000 U.S. households (U.S. DOE 2020). This means new imperatives exist in how we cool, maintain, and optimize our data center facilities.

Challenges of AI Cluster in Data Centers
There are three challenges for the Smart Computing Center: bandwidth increasing, latency reducing and power consumption minimizing. Therefore, 400G and 800G technologies based on multimode fiber will be heavily adopted.
In terms of networking challenges, GPU computing clusters require huge inter-server connections, but due to power and heat constraints, the number of servers per rack must be reduced. Due to large throughput demand between devices, more than 400G of bandwidth support is required, copper cable applications are reduced, and fiber optic cable applications are increased significantly.
In terms of energy consumption challenges, it requires about 40 kW to power GPU servers, and this is four or five times higher than typical server rack power, so that data centers built to lower power requirements will need to be upgraded or build GPU high-density rack areas exclusively.
In the ideal situation pictured by NVIDIA, all GPU servers in an AI cluster would be tightly coupled together. As with HPC, AI machine learning algorithms are extremely sensitive to link latency. NVIDIA’s internal statistics show that running a large training model spends 30 percent of its time on network latency and 70 percent of its time on computation. Since the cost of training a large model can be as high as $10 million, this network latency time represents a significant expense. Even saving 50 nanoseconds of latency or 10 meters of fiber optic line distance is significant on cost saving. Shorter link distances are even more necessary for time-sensitive inference data exchange; typically, AI clusters are limited to 100 meters of link.
Intelligent Computing Center Network Architecture
Almost all modern data centers, especially hyperscale data centers, use a folded CLOS architecture. All the leaf switches (LEAF) are connected to ridge switches (SPINE). In the mainframe room, servers are connected to the top-of-rack (ToR) switches, and then the ToR devices are connected to the leaf switches (LEAFs). Each server needs to be connected onto networks for switching, storage and etc.
As an example, NVIDIA’s latest GPU server DGXH100, featuring four 800G switch ports (running as eight 400GE), four 400GE storage ports, 1GE and 10GE management ports. As shown below, a DGXSuperPOD can contain 32 of these GPU servers connected to 18 switches. Each row would then have 384 400GE fiber links for the switch network and storage network, and 64 copper links for management purposes. Because of this all-connected architecture, the number of fiber links in the mainframe room will increase dramatically.
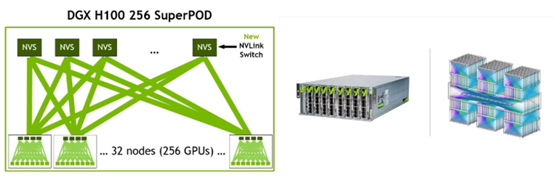
Data Center Considerations in Cabling and Cooling
Demands in connectivity of smart computing devices, represented by NVIDIA, have reached and exceeded 400G interface rates. Intelligent computing equipment has greatly promoted the commercialization of 400G, as well as the standardization 800G, hence press ahead industry development. High-performance fiber optic cables and high-density connectors are on next chapter.
Data center builders need to carefully consider which optical transceivers and cables to use for their AI clusters to minimize cost and power consumption. Of the total cost of fiber optic connectivity, the major cost is concentrated in the transceiver. The advantage of parallel fiber transceivers is the wavelength-division multiplexing (WDM) process doesn’t require optical multiplex units (OMU) and demultiplexers (DEMUX), hence reducing the cost and power consumption. These savings can cover the costs of replacing duplex cables with multicore fiber. For example, an 8-core 400G-DR4 transceiver is more cost effective than a 400G-FR4 transceiver with duplex (2 cores) fiber.
High-speed multimode transceivers consume a watt or two less power than single-mode transceivers. For example, a typical NVIDIA single AI cluster having 768 transceivers would save up to 1.5KW of power using a multimode fiber setup. This may seem insignificant compared to the 10KW consumed by each DGX H100, but for an AI cluster, you just can’t miss any chance in reducing power consumption. One tiny saving on power consumption can significantly reduce training costs and operating expenses.
Cooling, on the other hand, takes another significant portion in data center expenses. As 43% of total expenses goes into cooling and power provision, cost efficiency became a primary concern for enterprises. FBox’s liquid cooling data center adopts an integrated design of a liquid cooling unit and cooling tower, which provide efficient cooling without relying on water. FBox functions in modular design with features all built-in, so that spaces and costs were saved. Optimized liquid flow within single-phase immersion cooling decreases energy and water consumption while maximizing heat capture. In addition, extra heat energy can be recycled used for other purposes.
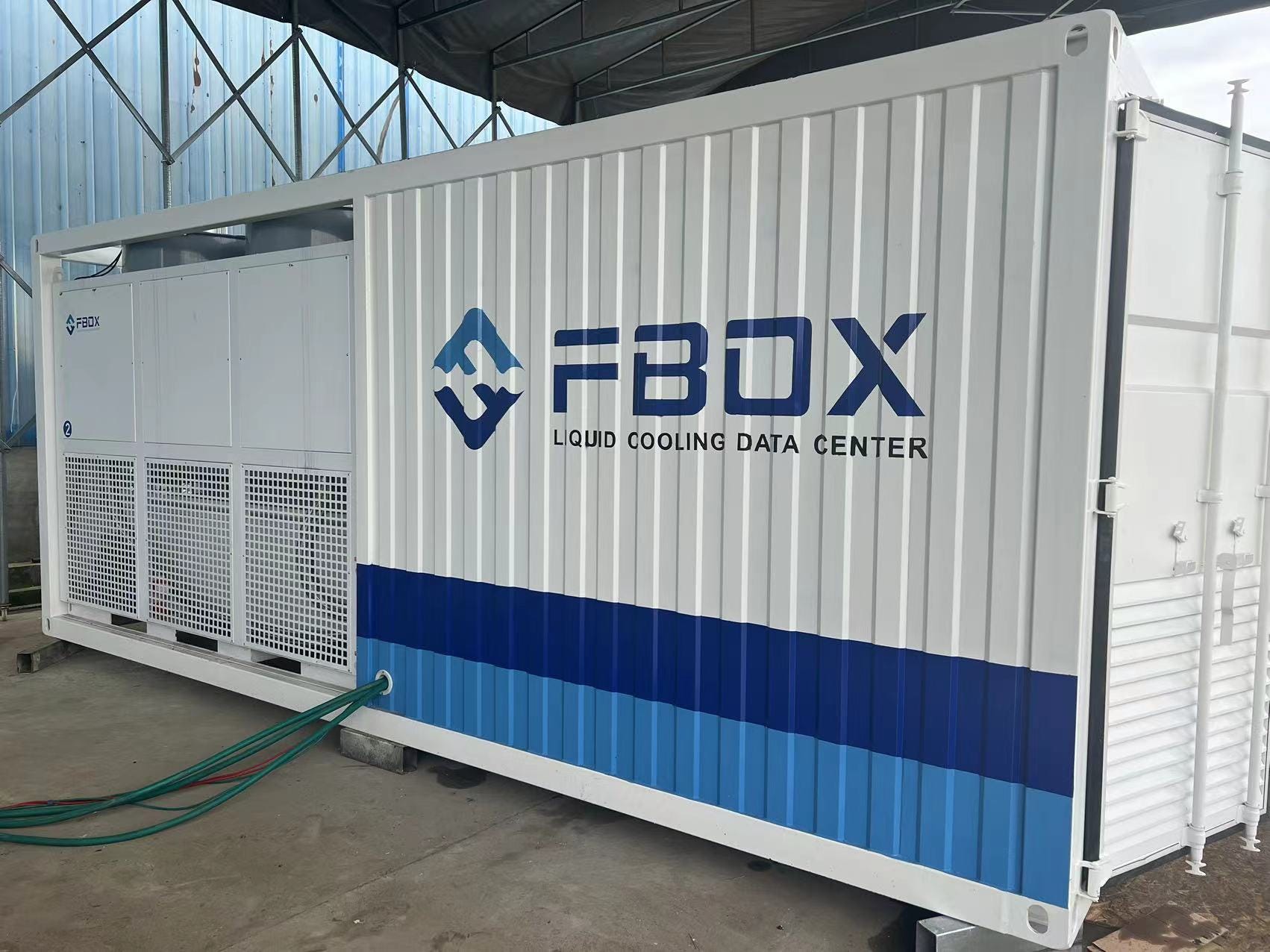
With the development of AIGC, large model training and inference require GPU support, which pushes the network connection of IQC to 400G and above application scenarios. Also, a high density, data driven data center will have higher requirements in cooling solutions, no doubt. Immersion liquid cooling can be the essential driver for your technology and business.
评论
发表评论